Background
Conservation and management of species and their habitats depends in part on knowing where those species and habitats occur both today as well as into the future. In the face of rapid climate change, being able to forecast regional- to global-scale changes in species distributions, species assemblages, and patterns of biodiversity is more critical than ever. Ecologists typically use statistical models as tools to make these types of forecasts, which are then used to inform management strategies. One common type of modeling approach used for this purpose is species distribution models (SDMs). To forecasts changes in species assemblages, individual SDMs are fitted and projected for each species, which are then aggregated, or stacked, to infer potential changes in community-level patterns. SDMs can be limited because they assume that species exist in isolation and independently of one another, which is most often not the case in the natural world.
Conversely, community-level models (CLMs) employ an ‘assemble and predict together’ strategy, which involves combining data from multiple species to simultaneously analyze and map patterns of biodiversity at the community level. CLMs can capture any process driving co-occurrence patterns, including shared climatic requirements, responses to unmeasured environmental variables, and possibly biotic interactions. By simultaneously modelling all observed species within a region of interest and incorporating co-occurrence data, CLMs may have the potential to predict species distributions and changes in community composition better than SDMs, especially for large climatic shifts and novel climate regimes. The potential greater transferability of CLMs to novel climates may also be beneficial considering the predicted emergence of no-analogue climates in the near future. However, these ideas have been untested and the relative ability of SDMs and CLMs to simulate the past emergence of no-analogue communities is unknown.
This work is the first to compare model predictions with observed species assemblages using head-to-head evaluations of five SDM algorithms and their direct CLM counterparts across longer time periods, as well as across climate change similar in magnitude to that expected this century. Using fossil pollen from sediment cores in eastern North America spanning the past 21,000 years, we performed the first comprehensive SDM and CLM model comparison across the large and rapid climate changes of the Late Quaternary to evaluate how these models may perform in predicting species distributions and assemblages under climate change.
Methods
We used paleoclimatic simulations and fossil-pollen records from the past 21,000 years since the Last Glacial Maximum (LGM – 21 kyr BP) to conduct a controlled comparison of five paired SDMs and CLMs, covering a wide range of model-class types. We examined taxon occurrence data for fossil-pollen from sediment cores collected in eastern North America and used paleoclimate simulations from the Community Climate System Model Version 3 (CCSM3) SynTrace transient simulation with seasonally averaged model outputs saved at a decadal time step from LGM to present. We used multiple metrics to evaluate the ability of the models to predict taxa distributions in order to examine the extent to which models can be reliably projected to new climatic regimes and no-analogue communities.
Results
SDMs and CLMs predicted species’ probability of occurrence similarly when projected to climatically similar time periods; however, when models were hind-casted or forecasted to climatically novel periods, CLMs outperformed SDMs. When models were projected to climatically similar time periods, both SDMs and CLMs were able to successfully discriminate between occupied and unoccupied sites for individual species, with SDMs tending to outperform CLMs under these circumstances. However, the opposite was true when models were projected to climatically dissimilar time periods. Both model types performed poorly; however, CLMs generally outperformed SDMs in these situations, especially when the models were fit with sparse calibration datasets. Additionally, CLMs did not over-fit training data, unlike SDMs.
In terms of predicting community composition, CLMs and stacked SDMs performed best when models were fitted in, and projected to, climatically similar time periods, with stacked SDMs tending to outperform CLMs. When models were fitted and projected to climatically novel time periods, the models exhibited equal performance, with SDMs hindcasting slightly better and CLMs forecasting slightly better.
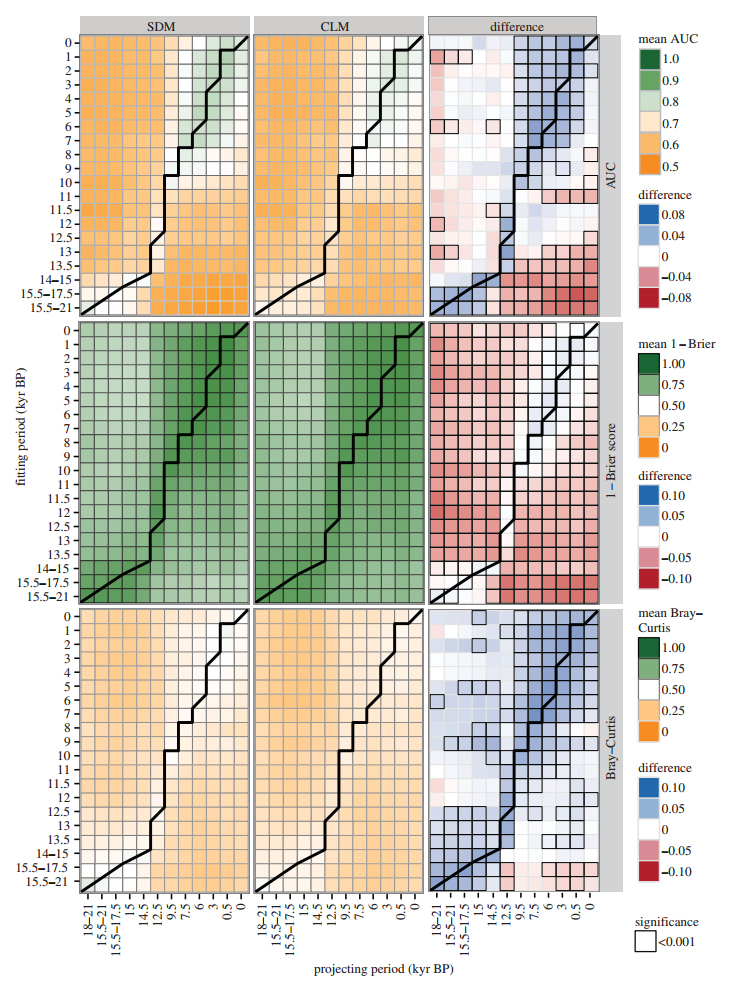
The graph to the right illustrates differences in performance between SDMs and CLMs. Mean AUC scores (top row), 1-Brier scores (middle row), and Bray-Curtis similarity (bottom row) of SDMs (left column) and CLMs (middle column) for each combination of fit in and projected to periods. Each square represents the average of all five modelling algorithms for all genera. Green shading indicates high performance and orange shading indicates low performance. The right column illustrates the differences between SDM and CLM scores across all five modelling algorithms and all genera. Blue shading indicates superior SDM performance while red shading indicates better CLM performance. Cells with black outlines indicate statistically significant differences between SDMs and CLMs. The thick solid black lines inidcate models that were fit and projected to the same time period and thus divide hindcasted models (above the line) from forecasted models (below the line).
View expanded version of figure.
Conclusions
The expected emergence of novel climates presents a major forecasting challenge for all models in order to accommodate the complex and shifting relationships between the environment, species distributions, and communities as the environment changes and communities reshuffle in response to those changes. We found that model transferability was low regardless of model type during periods of greatest climatic and compositional novelty. By simultaneously modelling all co-occurring taxa in a region, CLMs outperformed SDMs for the most novel climates or when forecasting from periods with sparse data. CLMs may better rise to this challenge because they identify primary climatic gradients driving biodiversity patterns and thereby buffer against idiosyncratic changes in individual species-environment relationships that can reduce SDM performance and they are more robust to small sample sizes. Because both SDMs and CLMs performed poorly when projected into novel climates and assemblages, the dependability and utility of using empirical models to project the future is questionable. Our work confirms that CLMS offer a modestly superior approach to SDMs for modelling the responses of species diversity and distributions to climate change. CLMs deserve greater attention, application, and examination than they have received to date.